Overview of the fitdistrplus package
Marie Laure Delignette Muller, Christophe Dutang
2025-01-12
Source:vignettes/fitdistrplus_vignette.Rmd
fitdistrplus_vignette.Rmd
Based on the article fitdistrplus: an R Package for Fitting Distributions (Marie Laure Delignette-Muller and Christophe Dutang, 2015, Journal of Statistical Software, DOI 10.18637/jss.v064.i04)
Keywords: probability distribution fitting, bootstrap, censored data, maximum likelihood, moment matching, quantile matching, maximum goodness-of-fit, distributions, R
1. Introduction
Fitting distributions to data is a very common task in statistics and
consists in choosing a probability distribution modelling the random
variable, as well as finding parameter estimates for that distribution.
This requires judgment and expertise and generally needs an iterative
process of distribution choice, parameter estimation, and quality of fit
assessment. In the R (R
Development Core Team 2013) package MASS
(Venables and Ripley
2010), maximum likelihood estimation is available via the
fitdistr
function; other steps of the fitting process can
be done using other R functions (Ricci 2005). In this paper, we present
the R package fitdistrplus (Delignette-Muller et al. 2014)
implementing several methods for fitting univariate parametric
distribution. A first objective in developing this package was to
provide R users a set of functions dedicated to help this overall
process.
The fitdistr
function estimates distribution parameters
by maximizing the likelihood function using the optim
function. No distinction between parameters with different roles (e.g.,
main parameter and nuisance parameter) is made, as this paper focuses on
parameter estimation from a general point-of-view. In some cases, other
estimation methods could be prefered, such as maximum goodness-of-fit
estimation (also called minimum distance estimation), as proposed in the
R package actuar with three different goodness-of-fit
distances (Dutang,
Goulet, and Pigeon 2008). While developping the
fitdistrplus package, a second objective was to
consider various estimation methods in addition to maximum likelihood
estimation (MLE). Functions were developped to enable moment matching
estimation (MME), quantile matching estimation (QME), and maximum
goodness-of-fit estimation (MGE) using eight different distances.
Moreover, the fitdistrplus package offers the
possibility to specify a user-supplied function for optimization, useful
in cases where classical optimization techniques, not included in
optim
, are more adequate.
In applied statistics, it is frequent to have to fit distributions to
censored data Commeau et al. (2012). The MASS
fitdistr
function does not enable maximum likelihood
estimation with this type of data. Some packages can be used to work
with censored data, especially survival data Jordan (2005), but
those packages generally focus on specific models, enabling the fit of a
restricted set of distributions. A third objective is thus to provide R
users a function to estimate univariate distribution parameters from
right-, left- and interval-censored data.
Few packages on CRAN provide estimation procedures for any
user-supplied parametric distribution and support different types of
data. The distrMod package (Kohl and Ruckdeschel 2010) provides
an object-oriented (S4) implementation of probability models and
includes distribution fitting procedures for a given minimization
criterion. This criterion is a user-supplied function which is
sufficiently flexible to handle censored data, yet not in a trivial way,
see Example M4 of the distrMod vignette. The fitting
functions MLEstimator
and MDEstimator
return
an S4 class for which a coercion method to class mle
is
provided so that the respective functionalities (e.g.,
confint
and logLik
) from package
stats4 are available, too. In
fitdistrplus, we chose to use the standard S3 class
system for its understanding by most R users. When designing the
fitdistrplus package, we did not forget to implement
generic functions also available for S3 classes. Finally, various other
packages provide functions to estimate the mode, the moments or the
L-moments of a distribution, see the reference manuals of
modeest, lmomco and
Lmoments packages.
The package is available from the Comprehensive R Archive Network at . The paper is organized as follows: Section 2 presents tools for fitting continuous distributions to classic non-censored data. Section 3 deals with other estimation methods and other types of data, before Section 4 concludes.
2. Fitting distributions to continuous non-censored data
2.1. Choice of candidate distributions
For illustrating the use of various functions of the
fitdistrplus package with continuous non-censored data,
we will first use a data set named groundbeef
which is
included in our package. This data set contains pointwise values of
serving sizes in grams, collected in a French survey, for ground beef
patties consumed by children under 5 years old. It was used in a
quantitative risk assessment published by Delignette-Muller and Cornu (2008).
## Loading required package: fitdistrplus
## Loading required package: MASS
## Loading required package: survival
## 'data.frame': 254 obs. of 1 variable:
## $ serving: num 30 10 20 24 20 24 40 20 50 30 ...
Before fitting one or more distributions to a data set, it is generally necessary to choose good candidates among a predefined set of distributions. This choice may be guided by the knowledge of stochastic processes governing the modeled variable, or, in the absence of knowledge regarding the underlying process, by the observation of its empirical distribution. To help the user in this choice, we developed functions to plot and characterize the empirical distribution.
First of all, it is common to start with plots of the empirical
distribution function and the histogram (or density plot), which can be
obtained with the plotdist
function of the
fitdistrplus package. This function provides two plots
(see Figure @ref(fig:figgroundbeef)): the left-hand plot is by default
the histogram on a density scale (or density plot of both, according to
values of arguments histo
and demp
) and the
right-hand plot the empirical cumulative distribution function
(CDF).
plotdist(groundbeef$serving, histo = TRUE, demp = TRUE)
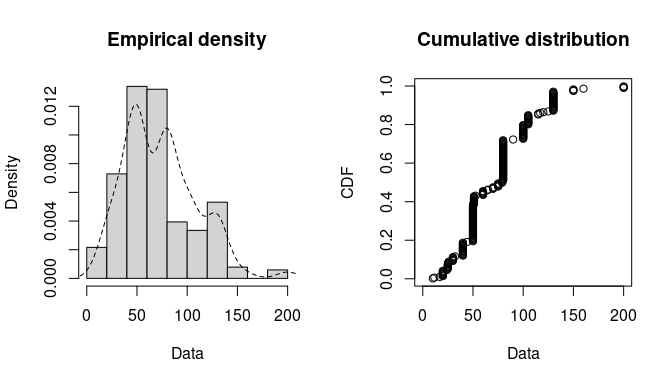
Histogram and CDF plots of an empirical distribution for a continuous
variable (serving size from the groundbeef
data set) as
provided by the plotdist
function.
In addition to empirical plots, descriptive statistics may help to choose candidates to describe a distribution among a set of parametric distributions. Especially the skewness and kurtosis, linked to the third and fourth moments, are useful for this purpose. A non-zero skewness reveals a lack of symmetry of the empirical distribution, while the kurtosis value quantifies the weight of tails in comparison to the normal distribution for which the kurtosis equals 3. The skewness and kurtosis and their corresponding unbiased estimator (Casella and Berger 2002) from a sample with observations are given by:
where , , denote empirical moments defined by , with the observations of variable and their mean value.
The descdist
function provides classical descriptive
statistics (minimum, maximum, median, mean, standard deviation),
skewness and kurtosis. By default, unbiased estimations of the three
last statistics are provided. Nevertheless, the argument
method
can be changed from "unbiased"
(default) to "sample"
to obtain them without correction for
bias. A skewness-kurtosis plot such as the one proposed by Cullen and Frey (1999) is provided by the
descdist
function for the empirical distribution (see
Figure @ref(fig:descgroundbeefplot) for the groundbeef
data
set). On this plot, values for common distributions are displayed in
order to help the choice of distributions to fit to data. For some
distributions (normal, uniform, logistic, exponential), there is only
one possible value for the skewness and the kurtosis. Thus, the
distribution is represented by a single point on the plot. For other
distributions, areas of possible values are represented, consisting in
lines (as for gamma and lognormal distributions), or larger areas (as
for beta distribution).
Skewness and kurtosis are known not to be robust. In order to take
into account the uncertainty of the estimated values of kurtosis and
skewness from data, a nonparametric bootstrap procedure (Efron and Tibshirani
1994) can be performed by using the argument
boot
. Values of skewness and kurtosis are computed on
bootstrap samples (constructed by random sampling with replacement from
the original data set) and reported on the skewness-kurtosis plot.
Nevertheless, the user needs to know that skewness and kurtosis, like
all higher moments, have a very high variance. This is a problem which
cannot be completely solved by the use of bootstrap. The
skewness-kurtosis plot should then be regarded as indicative only. The
properties of the random variable should be considered, notably its
expected value and its range, as a complement to the use of the
plotdist
and descdist
functions. Below is a
call to the descdist
function to describe the distribution
of the serving size from the groundbeef
data set and to
draw the corresponding skewness-kurtosis plot (see Figure
@ref(fig:descgroundbeefplot)). Looking at the results on this example
with a positive skewness and a kurtosis not far from 3, the fit of three
common right-skewed distributions could be considered, Weibull, gamma
and lognormal distributions.
descdist(groundbeef$serving, boot = 1000)
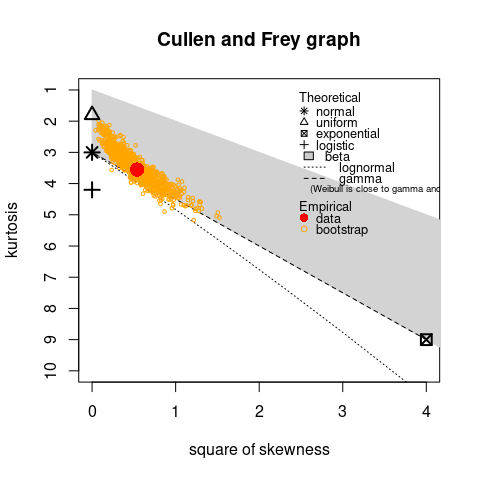
Skewness-kurtosis plot for a continuous variable (serving size from the
groundbeef
data set) as provided by the
descdist
function.
## summary statistics
## ------
## min: 10 max: 200
## median: 79
## mean: 73.65
## estimated sd: 35.88
## estimated skewness: 0.7353
## estimated kurtosis: 3.551
2.2. Fit of distributions by maximum likelihood estimation
Once selected, one or more parametric distributions
(with parameter
)
may be fitted to the data set, one at a time, using the
fitdist
function. Under the i.i.d. sample assumption,
distribution parameters
are by default estimated by maximizing the likelihood function defined
as:
with the observations of variable and the density function of the parametric distribution. The other proposed estimation methods are described in Section 3.1..
The fitdist
function returns an S3 object of class
fitdist
for which print
, summary
and plot
functions are provided. The fit of a distribution
using fitdist
assumes that the corresponding
d
, p
, q
functions (standing
respectively for the density, the distribution and the quantile
functions) are defined. Classical distributions are already defined in
that way in the stats package, e.g.,
dnorm
, pnorm
and qnorm
for the
normal distribution (see ?Distributions
). Others may be
found in various packages (see the CRAN task view: Probability
Distributions at ). Distributions not found in any package must be
implemented by the user as d
, p
,
q
functions. In the call to fitdist
, a
distribution has to be specified via the argument dist
either by the character string corresponding to its common root name
used in the names of d
, p
, q
functions (e.g., "norm"
for the normal distribution) or by
the density function itself, from which the root name is extracted
(e.g., dnorm
for the normal distribution). Numerical
results returned by the fitdist
function are (1) the
parameter estimates, (2) the estimated standard errors (computed from
the estimate of the Hessian matrix at the maximum likelihood solution),
(3) the loglikelihood, (4) Akaike and Bayesian information criteria (the
so-called AIC and BIC), and (5) the correlation matrix between parameter
estimates. Below is a call to the fitdist
function to fit a
Weibull distribution to the serving size from the
groundbeef
data set.
## Fitting of the distribution ' weibull ' by maximum likelihood
## Parameters :
## estimate Std. Error
## shape 2.186 0.1046
## scale 83.348 2.5269
## Loglikelihood: -1255 AIC: 2514 BIC: 2522
## Correlation matrix:
## shape scale
## shape 1.0000 0.3218
## scale 0.3218 1.0000
The plot of an object of class fitdist
provides four
classical goodness-of-fit plots (Cullen and Frey 1999) presented on
Figure @ref(fig:groundbeefcomp):
- a density plot representing the density function of the fitted distribution along with the histogram of the empirical distribution,
- a CDF plot of both the empirical distribution and the fitted distribution,
- a Q-Q plot representing the empirical quantiles (y-axis) against the theoretical quantiles (x-axis),
- a P-P plot representing the empirical distribution function evaluated at each data point (y-axis) against the fitted distribution function (x-axis).
For CDF, Q-Q and P-P plots, the probability plotting position is
defined by default using Hazen’s rule, with probability points of the
empirical distribution calculated as (1:n - 0.5)/n
, as
recommended by Blom (1959). This plotting position can be easily
changed (see the reference manual for details (Delignette-Muller et al. 2014)).
Unlike the generic plot
function, the
denscomp
, cdfcomp
, qqcomp
and
ppcomp
functions enable to draw separately each of these
four plots, in order to compare the empirical distribution and multiple
parametric distributions fitted on a same data set. These functions must
be called with a first argument corresponding to a list of objects of
class fitdist
, and optionally further arguments to
customize the plot (see the reference manual for lists of arguments that
may be specific to each plot (Delignette-Muller et al. 2014)). In
the following example, we compare the fit of a Weibull, a lognormal and
a gamma distributions to the groundbeef
data set (Figure
@ref(fig:groundbeefcomp)).
par(mfrow = c(2, 2), mar = c(4, 4, 2, 1))
fg <- fitdist(groundbeef$serving, "gamma")
fln <- fitdist(groundbeef$serving, "lnorm")
plot.legend <- c("Weibull", "lognormal", "gamma")
denscomp(list(fw, fln, fg), legendtext = plot.legend)
qqcomp(list(fw, fln, fg), legendtext = plot.legend)
cdfcomp(list(fw, fln, fg), legendtext = plot.legend)
ppcomp(list(fw, fln, fg), legendtext = plot.legend)
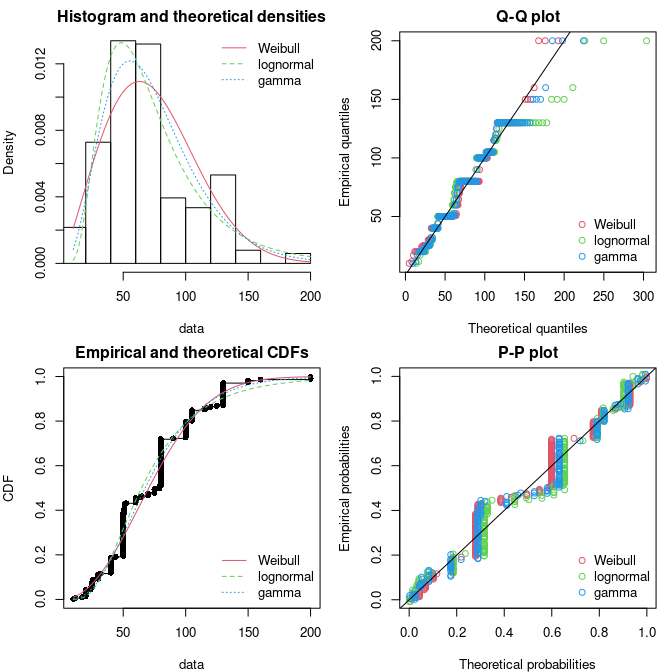
Four Goodness-of-fit plots for various distributions fitted to
continuous data (Weibull, gamma and lognormal distributions fitted to
serving sizes from the groundbeef
data set) as provided by
functions denscomp
, qqcomp
,
cdfcomp
and ppcomp
.
The density plot and the CDF plot may be considered as the basic classical goodness-of-fit plots. The two other plots are complementary and can be very informative in some cases. The Q-Q plot emphasizes the lack-of-fit at the distribution tails while the P-P plot emphasizes the lack-of-fit at the distribution center. In the present example (in Figure @ref(fig:groundbeefcomp)), none of the three fitted distributions correctly describes the center of the distribution, but the Weibull and gamma distributions could be prefered for their better description of the right tail of the empirical distribution, especially if this tail is important in the use of the fitted distribution, as it is in the context of food risk assessment.
The data set named endosulfan
will now be used to
illustrate other features of the fitdistrplus package.
This data set contains acute toxicity values for the organochlorine
pesticide endosulfan (geometric mean of LC50 ou EC50 values in
),
tested on Australian and non-Australian laboratory-species (Hose and Van den Brink
2004). In ecotoxicology, a lognormal or a loglogistic
distribution is often fitted to such a data set in order to characterize
the species sensitivity distribution (SSD) for a pollutant. A low
percentile of the fitted distribution, generally the 5% percentile, is
then calculated and named the hazardous concentration 5% (HC5). It is
interpreted as the value of the pollutant concentration protecting 95%
of the species (Posthuma, Suter, and Traas 2010).
But the fit of a lognormal or a loglogistic distribution to the whole
endosulfan
data set is rather bad (Figure
@ref(fig:fitendo)), especially due to a minority of very high values.
The two-parameter Pareto distribution and the three-parameter Burr
distribution (which is an extension of both the loglogistic and the
Pareto distributions) have been fitted. Pareto and Burr distributions
are provided in the package actuar. Until here, we did
not have to define starting values (in the optimization process) as
reasonable starting values are implicity defined within the
fitdist
function for most of the distributions defined in R
(see ?fitdist
for details). For other distributions like
the Pareto and the Burr distribution, initial values for the
distribution parameters have to be supplied in the argument
start
, as a named list with initial values for each
parameter (as they appear in the d
, p
,
q
functions). Having defined reasonable starting values1 various
distributions can be fitted and graphically compared. On this example,
the function cdfcomp
can be used to report CDF values in a
logscale so as to emphasize discrepancies on the tail of interest while
defining an HC5 value (Figure @ref(fig:fitendo)).
## Loading required package: actuar
##
## Attaching package: 'actuar'
## The following objects are masked from 'package:stats':
##
## sd, var
## The following object is masked from 'package:grDevices':
##
## cm
data("endosulfan")
ATV <- endosulfan$ATV
fendo.ln <- fitdist(ATV, "lnorm")
fendo.ll <- fitdist(ATV, "llogis", start = list(shape = 1, scale = 500))
fendo.P <- fitdist(ATV, "pareto", start = list(shape = 1, scale = 500))
fendo.B <- fitdist(ATV, "burr", start = list(shape1 = 0.3, shape2 = 1, rate = 1))
cdfcomp(list(fendo.ln, fendo.ll, fendo.P, fendo.B), xlogscale = TRUE,
ylogscale = TRUE, legendtext = c("lognormal", "loglogistic", "Pareto", "Burr"))
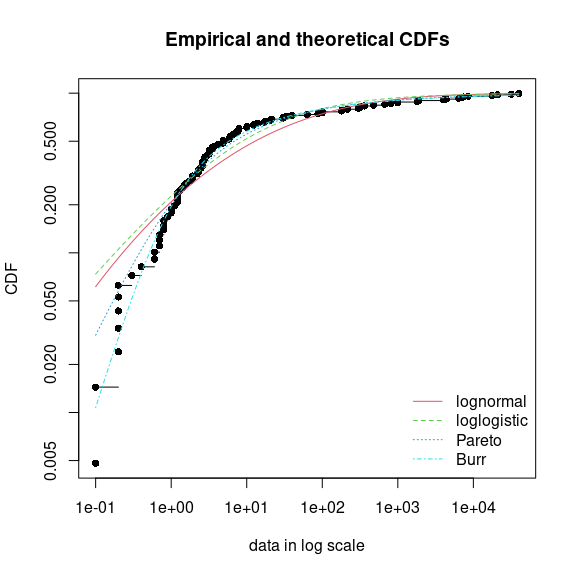
CDF plot to compare the fit of four distributions to acute toxicity
values of various organisms for the organochlorine pesticide endosulfan
(endosulfan
data set) as provided by the
cdfcomp
function, with CDF values in a logscale to
emphasize discrepancies on the left tail.
None of the fitted distribution correctly describes the right tail
observed in the data set, but as shown in Figure @ref(fig:fitendo), the
left-tail seems to be better described by the Burr distribution. Its use
could then be considered to estimate the HC5 value as the 5% quantile of
the distribution. This can be easily done using the
quantile
generic function defined for an object of class
fitdist
. Below is this calculation together with the
calculation of the empirical quantile for comparison.
quantile(fendo.B, probs = 0.05)
## Estimated quantiles for each specified probability (non-censored data)
## p=0.05
## estimate 0.2939
quantile(ATV, probs = 0.05)
## 5%
## 0.2
In addition to the ecotoxicology context, the quantile
generic function is also attractive in the actuarial-financial context.
In fact, the value-at-risk
is defined as the
-quantile
of the loss distribution and can be computed with quantile
on a fitdist
object.
The computation of different goodness-of-fit statistics is proposed
in the fitdistrplus package in order to further compare
fitted distributions. The purpose of goodness-of-fit statistics aims to
measure the distance between the fitted parametric distribution and the
empirical distribution: e.g., the distance between the fitted cumulative
distribution function
and the empirical distribution function
.
When fitting continuous distributions, three goodness-of-fit statistics
are classicaly considered: Cramer-von Mises, Kolmogorov-Smirnov and
Anderson-Darling statistics (D’Agostino and Stephens 1986). Naming
the
observations of a continuous variable
arranged in an ascending order, Table @ref(tab:tabKSCvMAD) gives the
definition and the empirical estimate of the three considered
goodness-of-fit statistics. They can be computed using the function
gofstat
as defined by Stephens (D’Agostino and Stephens 1986).
gofstat(list(fendo.ln, fendo.ll, fendo.P, fendo.B),
fitnames = c("lnorm", "llogis", "Pareto", "Burr"))
## Goodness-of-fit statistics
## lnorm llogis Pareto Burr
## Kolmogorov-Smirnov statistic 0.1672 0.1196 0.08488 0.06155
## Cramer-von Mises statistic 0.6374 0.3827 0.13926 0.06803
## Anderson-Darling statistic 3.4721 2.8316 0.89206 0.52393
##
## Goodness-of-fit criteria
## lnorm llogis Pareto Burr
## Akaike's Information Criterion 1069 1069 1048 1046
## Bayesian Information Criterion 1074 1075 1053 1054
Statistic | General formula | Computational formula |
---|---|---|
Kolmogorov-Smirnov (KS) | with and | |
Cramer-von Mises (CvM) | ||
Anderson-Darling (AD) |
where
As giving more weight to distribution tails, the Anderson-Darling statistic is of special interest when it matters to equally emphasize the tails as well as the main body of a distribution. This is often the case in risk assessment Vose (2010). For this reason, this statistics is often used to select the best distribution among those fitted. Nevertheless, this statistics should be used cautiously when comparing fits of various distributions. Keeping in mind that the weighting of each CDF quadratic difference depends on the parametric distribution in its definition (see Table @ref(tab:tabKSCvMAD)), Anderson-Darling statistics computed for several distributions fitted on a same data set are theoretically difficult to compare. Moreover, such a statistic, as Cramer-von Mises and Kolmogorov-Smirnov ones, does not take into account the complexity of the model (i.e., parameter number). It is not a problem when compared distributions are characterized by the same number of parameters, but it could systematically promote the selection of the more complex distributions in the other case. Looking at classical penalized criteria based on the loglikehood (AIC, BIC) seems thus also interesting, especially to discourage overfitting.
In the previous example, all the goodness-of-fit statistics based on the CDF distance are in favor of the Burr distribution, the only one characterized by three parameters, while AIC and BIC values respectively give the preference to the Burr distribution or the Pareto distribution. The choice between these two distributions seems thus less obvious and could be discussed. Even if specifically recommended for discrete distributions, the Chi-squared statistic may also be used for continuous distributions (see Section 3.3. and the reference manual for examples (Delignette-Muller et al. 2014)).
2.3. Uncertainty in parameter estimates
The uncertainty in the parameters of the fitted distribution can be
estimated by parametric or nonparametric bootstraps using the
boodist
function for non-censored data (Efron and Tibshirani
1994). This function returns the bootstrapped values of
parameters in an S3 class object which can be plotted to visualize the
bootstrap region. The medians and the 95% confidence intervals of
parameters (2.5 and 97.5 percentiles) are printed in the summary. When
inferior to the whole number of iterations (due to lack of convergence
of the optimization algorithm for some bootstrapped data sets), the
number of iterations for which the estimation converges is also printed
in the summary.
The plot of an object of class bootdist
consists in a
scatterplot or a matrix of scatterplots of the bootstrapped values of
parameters providing a representation of the joint uncertainty
distribution of the fitted parameters. Below is an example of the use of
the bootdist
function with the previous fit of the Burr
distribution to the endosulfan
data set (Figure
@ref(fig:bootstrap)).
## Parametric bootstrap medians and 95% percentile CI
## Median 2.5% 97.5%
## shape1 0.1983 0.09283 0.3606
## shape2 1.5863 1.05306 3.0629
## rate 1.4907 0.70828 2.7775
plot(bendo.B)
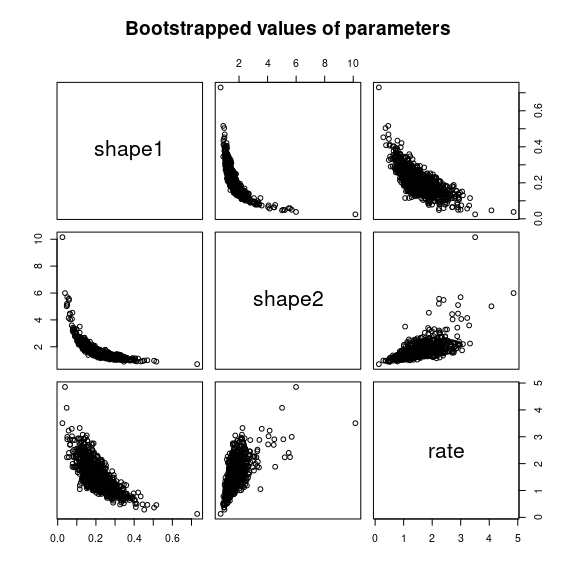
Bootstrappped values of parameters for a fit of the Burr distribution
characterized by three parameters (example on the
endosulfan
data set) as provided by the plot of an object
of class bootdist
.
Bootstrap samples of parameter estimates are useful especially to calculate confidence intervals on each parameter of the fitted distribution from the marginal distribution of the bootstraped values. It is also interesting to look at the joint distribution of the bootstraped values in a scatterplot (or a matrix of scatterplots if the number of parameters exceeds two) in order to understand the potential structural correlation between parameters (see Figure @ref(fig:bootstrap)).
The use of the whole bootstrap sample is also of interest in the risk assessment field. Its use enables the characterization of uncertainty in distribution parameters. It can be directly used within a second-order Monte Carlo simulation framework, especially within the package mc2d (Pouillot, Delignette-Muller, and Denis 2011). One could refer to Pouillot and Delignette-Muller (2010) for an introduction to the use of mc2d and fitdistrplus packages in the context of quantitative risk assessment.
The bootstrap method can also be used to calculate confidence
intervals on quantiles of the fitted distribution. For this purpose, a
generic quantile
function is provided for class
bootdist
. By default, 95% percentiles bootstrap confidence
intervals of quantiles are provided. Going back to the previous example
from ecotoxicolgy, this function can be used to estimate the uncertainty
associated to the HC5 estimation, for example from the previously fitted
Burr distribution to the endosulfan
data set.
quantile(bendo.B, probs = 0.05)
## (original) estimated quantiles for each specified probability (non-censored data)
## p=0.05
## estimate 0.2939
## Median of bootstrap estimates
## p=0.05
## estimate 0.2994
##
## two-sided 95 % CI of each quantile
## p=0.05
## 2.5 % 0.1792
## 97.5 % 0.4999
3. Advanced topics
3.1. Alternative methods for parameter estimation
This subsection focuses on alternative estimation methods. One of the alternative for continuous distributions is the maximum goodness-of-fit estimation method also called minimum distance estimation method Dutang, Goulet, and Pigeon (2008). In this package this method is proposed with eight different distances: the three classical distances defined in Table @ref(tab:tabKSCvMAD), or one of the variants of the Anderson-Darling distance proposed by Luceno (2006) and defined in Table @ref(tab:modifiedAD). The right-tail AD gives more weight to the right-tail, the left-tail AD gives more weight only to the left tail. Either of the tails, or both of them, can receive even larger weights by using second order Anderson-Darling Statistics.
Statistic | General formula | Computational formula |
---|---|---|
Right-tail AD (ADR) | ||
Left-tail AD (ADL) | ||
Right-tail AD 2nd order (AD2R) | ||
Left-tail AD 2nd order (AD2L) | ||
AD 2nd order (AD2) |
where and
To fit a distribution by maximum goodness-of-fit estimation, one
needs to fix the argument method
to mge
in the
call to fitdist
and to specify the argument
gof
coding for the chosen goodness-of-fit distance. This
function is intended to be used only with continuous non-censored
data.
Maximum goodness-of-fit estimation may be useful to give more weight
to data at one tail of the distribution. In the previous example from
ecotoxicology, we used a non classical distribution (the Burr
distribution) to correctly fit the empirical distribution especially on
its left tail. In order to correctly estimate the
5
percentile, we could also consider the fit of the classical lognormal
distribution, but minimizing a goodness-of-fit distance giving more
weight to the left tail of the empirical distribution. In what follows,
the left tail Anderson-Darling distances of first or second order are
used to fit a lognormal to endosulfan
data set (see Figure
@ref(fig:plotfitMGE)).
fendo.ln.ADL <- fitdist(ATV, "lnorm", method = "mge", gof = "ADL")
fendo.ln.AD2L <- fitdist(ATV, "lnorm", method = "mge", gof = "AD2L")
cdfcomp(list(fendo.ln, fendo.ln.ADL, fendo.ln.AD2L),
xlogscale = TRUE, ylogscale = TRUE,
main = "Fitting a lognormal distribution",
xlegend = "bottomright",
legendtext = c("MLE", "Left-tail AD", "Left-tail AD 2nd order"))
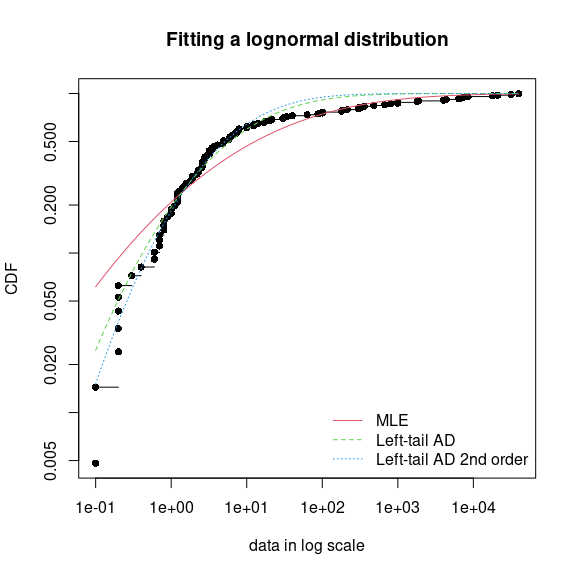
Comparison of a lognormal distribution fitted by MLE and by MGE using
two different goodness-of-fit distances: left-tail Anderson-Darling and
left-tail Anderson Darling of second order (example with the
endosulfan
data set) as provided by the
cdfcomp
function, with CDF values in a logscale to
emphasize discrepancies on the left tail.
Comparing the 5% percentiles (HC5) calculated using these three fits to the one calculated from the MLE fit of the Burr distribution, we can observe, on this example, that fitting the lognormal distribution by maximizing left tail Anderson-Darling distances of first or second order enables to approach the value obtained by fitting the Burr distribution by MLE.
(HC5.estimates <- c(
empirical = as.numeric(quantile(ATV, probs = 0.05)),
Burr = as.numeric(quantile(fendo.B, probs = 0.05)$quantiles),
lognormal_MLE = as.numeric(quantile(fendo.ln, probs = 0.05)$quantiles),
lognormal_AD2 = as.numeric(quantile(fendo.ln.ADL, probs = 0.05)$quantiles),
lognormal_AD2L = as.numeric(quantile(fendo.ln.AD2L, probs = 0.05)$quantiles)))
## empirical Burr lognormal_MLE lognormal_AD2 lognormal_AD2L
## 0.20000 0.29393 0.07259 0.19591 0.25877
The moment matching estimation (MME) is another method commonly used to fit parametric distributions (Vose 2010). MME consists in finding the value of the parameter that equalizes the first theoretical raw moments of the parametric distribution to the corresponding empirical raw moments as in Equation @ref(eq:eq4):
for , with the number of parameters to estimate and the observations of variable . For moments of order greater than or equal to 2, it may also be relevant to match centered moments. Therefore, we match the moments given in Equation @ref(eq:eq5):
where
denotes the empirical centered moments. This method can be performed by
setting the argument method
to "mme"
in the
call to fitdist
. The estimate is computed by a closed-form
formula for the following distributions: normal, lognormal, exponential,
Poisson, gamma, logistic, negative binomial, geometric, beta and uniform
distributions. In this case, for distributions characterized by one
parameter (geometric, Poisson and exponential), this parameter is simply
estimated by matching theoretical and observed means, and for
distributions characterized by two parameters, these parameters are
estimated by matching theoretical and observed means and variances (Vose 2010). For other
distributions, the equation of moments is solved numerically using the
optim
function by minimizing the sum of squared differences
between observed and theoretical moments (see the
fitdistrplus reference manual for technical details
(Delignette-Muller et
al. 2014)).
A classical data set from the Danish insurance industry published in
McNeil (1997)
will be used to illustrate this method. In
fitdistrplus, the data set is stored in
danishuni
for the univariate version and contains the loss
amounts collected at Copenhagen Reinsurance between 1980 and 1990. In
actuarial science, it is standard to consider positive heavy-tailed
distributions and have a special focus on the right-tail of the
distributions. In this numerical experiment, we choose classic actuarial
distributions for loss modelling: the lognormal distribution and the
Pareto type II distribution (Klugman, Panjer, and Willmot
2009).
The lognormal distribution is fitted to danishuni
data
set by matching moments implemented as a closed-form formula. On the
left-hand graph of Figure @ref(fig:danishmme), the fitted distribution
functions obtained using the moment matching estimation (MME) and
maximum likelihood estimation (MLE) methods are compared. The MME method
provides a more cautious estimation of the insurance risk as the
MME-fitted distribution function (resp. MLE-fitted) underestimates
(overestimates) the empirical distribution function for large values of
claim amounts.
## 'data.frame': 2167 obs. of 2 variables:
## $ Date: Date, format: "1980-01-03" "1980-01-04" ...
## $ Loss: num 1.68 2.09 1.73 1.78 4.61 ...
fdanish.ln.MLE <- fitdist(danishuni$Loss, "lnorm")
fdanish.ln.MME <- fitdist(danishuni$Loss, "lnorm", method = "mme", order = 1:2)
require("actuar")
fdanish.P.MLE <- fitdist(danishuni$Loss, "pareto", start = list(shape = 10, scale = 10),
lower = 2+1e-6, upper = Inf)
## Warning in cov2cor(varcovar): diag(V) had non-positive or NA entries; the
## non-finite result may be dubious
## Warning in sqrt(diag(varcovar)): NaNs produced
memp <- function(x, order) mean(x^order)
fdanish.P.MME <- fitdist(danishuni$Loss, "pareto", method = "mme", order = 1:2, memp = "memp",
start = list(shape = 10, scale = 10), lower = c(2+1e-6, 2+1e-6),
upper = c(Inf, Inf))
## Warning in cov2cor(varcovar): diag(V) had non-positive or NA entries; the
## non-finite result may be dubious
par(mfrow = c(1, 2))
cdfcomp(list(fdanish.ln.MLE, fdanish.ln.MME), legend = c("lognormal MLE", "lognormal MME"),
main = "Fitting a lognormal distribution", xlogscale = TRUE, datapch = 20)
cdfcomp(list(fdanish.P.MLE, fdanish.P.MME), legend = c("Pareto MLE", "Pareto MME"),
main = "Fitting a Pareto distribution", xlogscale = TRUE, datapch = 20)
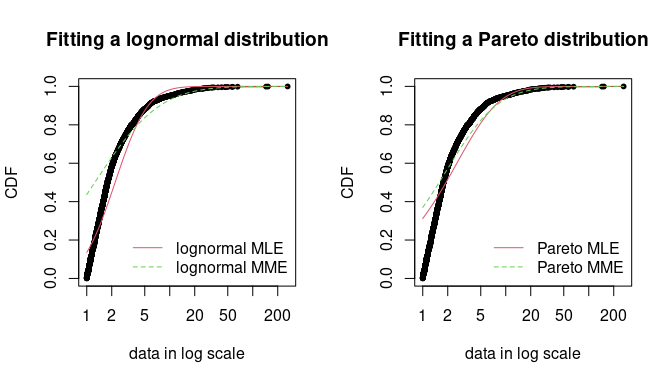
Comparison between MME and MLE when fitting a lognormal or a Pareto
distribution to loss data from the danishuni
data set.
In a second time, a Pareto distribution, which gives more weight to the right-tail of the distribution, is fitted. As the lognormal distribution, the Pareto has two parameters, which allows a fair comparison.
We use the implementation of the actuar package
providing raw and centered moments for that distribution (in addition to
d
, p
, q
and r
functions (Goulet
2012). Fitting a heavy-tailed distribution for which the
first and the second moments do not exist for certain values of the
shape parameter requires some cautiousness. This is carried out by
providing, for the optimization process, a lower and an upper bound for
each parameter. The code below calls the L-BFGS-B optimization method in
optim
, since this quasi-Newton allows box constraints 2. We choose
match moments defined in Equation @ref(eq:eq4), and so a function for
computing the empirical raw moment (called memp
in our
example) is passed to fitdist
. For two-parameter
distributions (i.e.,
),
Equations @ref(eq:eq4) and @ref(eq:eq5) are equivalent.
gofstat(list(fdanish.ln.MLE, fdanish.P.MLE, fdanish.ln.MME, fdanish.P.MME),
fitnames = c("lnorm.mle", "Pareto.mle", "lnorm.mme", "Pareto.mme"))
## Goodness-of-fit statistics
## lnorm.mle Pareto.mle lnorm.mme Pareto.mme
## Kolmogorov-Smirnov statistic 0.1375 0.3124 0.4368 0.37
## Cramer-von Mises statistic 14.7911 37.7166 88.9503 55.43
## Anderson-Darling statistic 87.1933 208.3143 416.2567 281.58
##
## Goodness-of-fit criteria
## lnorm.mle Pareto.mle lnorm.mme Pareto.mme
## Akaike's Information Criterion 8120 9250 9792 9409
## Bayesian Information Criterion 8131 9261 9803 9420
As shown on Figure @ref(fig:danishmme), MME and MLE fits are far less distant (when looking at the right-tail) for the Pareto distribution than for the lognormal distribution on this data set. Furthermore, for these two distributions, the MME method better fits the right-tail of the distribution from a visual point of view. This seems logical since empirical moments are influenced by large observed values. In the previous traces, we gave the values of goodness-of-fit statistics. Whatever the statistic considered, the MLE-fitted lognormal always provides the best fit to the observed data.
Maximum likelihood and moment matching estimations are certainly the most commonly used method for fitting distributions (Cullen and Frey 1999). Keeping in mind that these two methods may produce very different results, the user should be aware of its great sensitivity to outliers when choosing the moment matching estimation. This may be seen as an advantage in our example if the objective is to better describe the right tail of the distribution, but it may be seen as a drawback if the objective is different.
Fitting of a parametric distribution may also be done by matching theoretical quantiles of the parametric distributions (for specified probabilities) against the empirical quantiles (Tse 2009). The equality of theoretical and empirical quantiles is expressed by Equation @ref(eq:eq6) below, which is very similar to Equations @ref(eq:eq4) and @ref(eq:eq5):
for , with the number of parameters to estimate (dimension of if there is no fixed parameters) and the empirical quantiles calculated from data for specified probabilities .
Quantile matching estimation (QME) is performed by setting the
argument method
to "qme"
in the call to
fitdist
and adding an argument probs
defining
the probabilities for which the quantile matching is performed (see
Figure @ref(fig:danishqme)). The length of this vector must be equal to
the number of parameters to estimate (as the vector of moment orders for
MME). Empirical quantiles are computed using the quantile
function of the stats package using type=7
by default (see ?quantile
and Hyndman and Fan (1996)). But the type of quantile can
be easily changed by using the qty
argument in the call to
the qme
function.
The quantile matching is carried out numerically, by minimizing the sum
of squared differences between observed and theoretical quantiles.
fdanish.ln.QME1 <- fitdist(danishuni$Loss, "lnorm", method = "qme", probs = c(1/3, 2/3))
fdanish.ln.QME2 <- fitdist(danishuni$Loss, "lnorm", method = "qme", probs = c(8/10, 9/10))
cdfcomp(list(fdanish.ln.MLE, fdanish.ln.QME1, fdanish.ln.QME2),
legend = c("MLE", "QME(1/3, 2/3)", "QME(8/10, 9/10)"),
main = "Fitting a lognormal distribution", xlogscale = TRUE, datapch = 20)
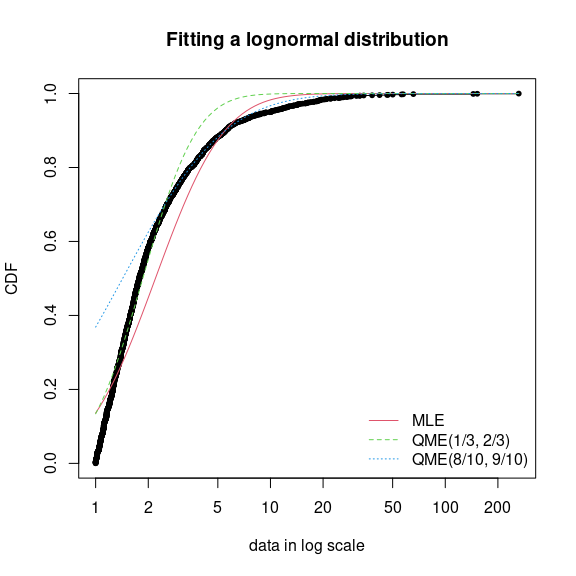
Comparison between QME and MLE when fitting a lognormal distribution to
loss data from the danishuni
data set.
Above is an example of fitting of a lognormal distribution to `danishuni} data set by matching probabilities and . As expected, the second QME fit gives more weight to the right-tail of the distribution. Compared to the maximum likelihood estimation, the second QME fit best suits the right-tail of the distribution, whereas the first QME fit best models the body of the distribution. The quantile matching estimation is of particular interest when we need to focus around particular quantiles, e.g., in the Solvency II insurance context or for the HC5 estimation in the ecotoxicology context.
3.2. Customization of the optimization algorithm
Each time a numerical minimization is carried out in the
fitdistrplus
package, the optim
function of
the stats package is used by default with the
Nelder-Mead
method for distributions characterized by more
than one parameter and the BFGS
method for distributions
characterized by only one parameter. Sometimes the default algorithm
fails to converge. It is then interesting to change some options of the
optim
function or to use another optimization function than
optim
to minimize the objective function. The argument
optim.method
can be used in the call to
fitdist
or fitdistcens
. It will internally be
passed to mledist
, mmedist
,
mgedist
or qmedist
, and to optim
(see ?optim
for details about the different algorithms
available).
Even if no error is raised when computing the optimization, changing
the algorithm is of particular interest to enforce bounds on some
parameters. For instance, a volatility parameter
is strictly positive
and a probability parameter
lies in
.
This is possible by using arguments lower
and/or
upper
, for which their use automatically forces
optim.method="L-BFGS-B"
.
Below are examples of fits of a gamma distribution
to the groundbeef
data set with various algorithms. Note
that the conjugate gradient algorithm (CG
) needs far more
iterations to converge (around 2500 iterations) compared to other
algorithms (converging in less than 100 iterations).
data("groundbeef")
fNM <- fitdist(groundbeef$serving, "gamma", optim.method = "Nelder-Mead")
fBFGS <- fitdist(groundbeef$serving, "gamma", optim.method = "BFGS")
fSANN <- fitdist(groundbeef$serving, "gamma", optim.method = "SANN")
fCG <- try(fitdist(groundbeef$serving, "gamma", optim.method = "CG",
control = list(maxit = 10000)))
if(inherits(fCG, "try-error")) {fCG <- list(estimate = NA)}
It is also possible to use another function than optim
to minimize the objective function by specifying by the argument
custom.optim
in the call to fitdist
. It may be
necessary to customize this optimization function to meet the following
requirements. (1) custom.optim
function must have the
following arguments: fn
for the function to be optimized
and par
for the initialized parameters. (2)
custom.optim
should carry out a MINIMIZATION and must
return the following components: par
for the estimate,
convergence
for the convergence code,
value=fn(par)
and hessian
. Below is an example
of code written to wrap the genoud
function from the
rgenoud package in order to respect our optimization
``template’’. The rgenoud package implements the
genetic (stochastic) algorithm.
mygenoud <- function(fn, par, ...)
{
require("rgenoud")
res <- genoud(fn, starting.values = par, ...)
standardres <- c(res, convergence = 0)
return(standardres)
}
The customized optimization function can then be passed as the
argument custom.optim
in the call to fitdist
or fitdistcens
. The following code can for example be used
to fit a gamma distribution to the groundbeef
data set.
Note that in this example various arguments are also passed from
fitdist
to genoud
: nvars
,
Domains
, boundary.enforcement
,
print.level
and hessian
. The code below
compares all the parameter estimates
(,
)
by the different algorithms: shape
and rate
parameters are relatively similar on this example, roughly 4.00 and
0.05, respectively.
fgenoud <- mledist(groundbeef$serving, "gamma", custom.optim = mygenoud, nvars = 2,
max.generations = 10, Domains = cbind(c(0, 0), c(10, 10)),
boundary.enforcement = 1, hessian = TRUE, print.level = 0, P9 = 10)
## Loading required package: rgenoud
## ## rgenoud (Version 5.9-0.11, Build Date: 2024-10-03)
## ## See http://sekhon.berkeley.edu/rgenoud for additional documentation.
## ## Please cite software as:
## ## Walter Mebane, Jr. and Jasjeet S. Sekhon. 2011.
## ## ``Genetic Optimization Using Derivatives: The rgenoud package for R.''
## ## Journal of Statistical Software, 42(11): 1-26.
## ##
cbind(NM = fNM$estimate, BFGS = fBFGS$estimate, SANN = fSANN$estimate, CG = fCG$estimate,
fgenoud = fgenoud$estimate)
## NM BFGS SANN CG fgenoud
## shape 4.00956 4.21184 3.93636 4.03958 4.00834
## rate 0.05444 0.05719 0.05366 0.05486 0.05443
3.3. Fitting distributions to other types of data
This section was modified since the publication of this vignette in the Journal of Statistical Software in order to include new goodness-of-fit plots for censored and discrete data.
Analytical methods often lead to semi-quantitative results which are referred to as censored data. Observations only known to be under a limit of detection are left-censored data. Observations only known to be above a limit of quantification are right-censored data. Results known to lie between two bounds are interval-censored data. These two bounds may correspond to a limit of detection and a limit of quantification, or more generally to uncertainty bounds around the observation. Right-censored data are also commonly encountered with survival data (Klein and Moeschberger 2003). A data set may thus contain right-, left-, or interval-censored data, or may be a mixture of these categories, possibly with different upper and lower bounds. Censored data are sometimes excluded from the data analysis or replaced by a fixed value, which in both cases may lead to biased results. A more recommended approach to correctly model such data is based upon maximum likelihood Helsel (2005).
Censored data may thus contain left-censored, right-censored and
interval-censored values, with several lower and upper bounds. Before
their use in package fitdistrplus, such data must be
coded into a dataframe with two columns, respectively named
left
and right
, describing each observed value
as an interval. The left
column contains either
NA
for left censored observations, the left bound of the
interval for interval censored observations, or the observed value for
non-censored observations. The right
column contains either
NA
for right censored observations, the right bound of the
interval for interval censored observations, or the observed value for
non-censored observations. To illustrate the use of package
fitdistrplus to fit distributions to censored continous
data, we will use another data set from ecotoxicology, included in our
package and named salinity
. This data set contains acute
salinity tolerance (LC50 values in electrical conductivity,
.)
of riverine macro-invertebrates taxa from the southern Murray-Darling
Basin in Central Victoria, Australia (Kefford et al. 2007).
## 'data.frame': 108 obs. of 2 variables:
## $ left : num 20 20 20 20 20 21.5 15 20 23.7 25 ...
## $ right: num NA NA NA NA NA 21.5 30 25 23.7 NA ...
Using censored data such as those coded in the
salinity} data set, the empirical distribution can be plotted using the
plotdistcens}
function. In older versions of the package, by default this function
used the Expectation-Maximization approach of Turnbull (1974) to
compute the overall empirical cdf curve with optional confidence
intervals, by calls to survfit
and
plot.survfit
functions from the survival
package. Even if this representation is always available (by fixing the
argument NPMLE.method
to
"Turnbull.middlepoints"
), now the default plot of the
empirical cumulative distribution function (ECDF) explicitly represents
the regions of non uniqueness of the NPMLE ECDF. The default computation
of those regions of non uniqueness and their associated masses uses the
non parametric maximum likelihood estimation (NPMLE) approach developped
by Wang Wang and Fani (2018).
Figure @ref(fig:cdfcompcens) shows on the top left the new plot of data
together with two fitted distributions. Grey filled rectangles in such a
plot represent the regions of non uniqueness of the NPMLE ECDF.
A less rigorous but sometimes more illustrative plot can be obtained
by fixing the argument NPMLE
to FALSE
in the
call to plotdistcens
(see Figure @ref(fig:plotsalinity2)
for an example and the help page of Function plotdistcens
for details). This plot enables to see the real nature of censored data,
as points and intervals, but the difficulty in building such a plot is
to define a relevant ordering of observations.
plotdistcens(salinity, NPMLE = FALSE)
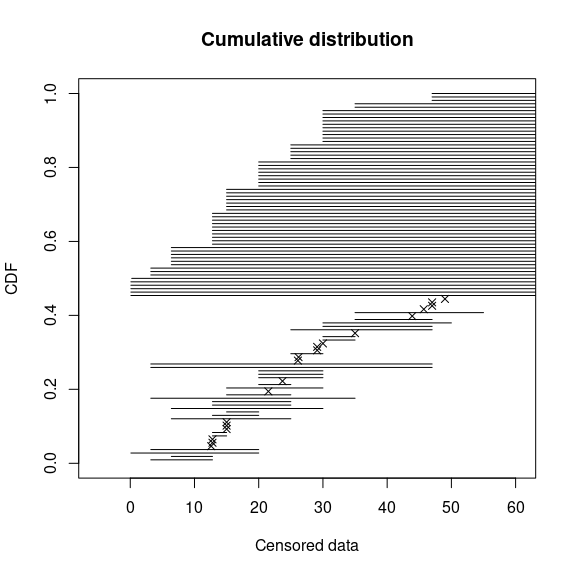
Simple plot of censored raw data (72-hour acute salinity tolerance of
riverine macro-invertebrates from the salinity
data set) as
ordered points and intervals.
As for non censored data, one or more parametric distributions can be
fitted to the censored data set, one at a time, but using in this case
the fitdistcens
function. This function estimates the
vector of distribution parameters
by maximizing the likelihood for censored data defined as:
$$\begin{equation} L(\theta) = \prod_{i=1}^{N_{nonC}} f(x_{i}|\theta)\times \prod_{j=1}^{N_{leftC}} F(x^{upper}_{j}|\theta) \\ \times \prod_{k=1}^{N_{rightC}} (1- F(x^{lower}_{k}|\theta))\times \prod_{m=1}^{N_{intC}} (F(x^{upper}_{m}|\theta)- F(x^{lower}_{j}|\theta))(\#eq:eq7) \end{equation}$$
with the non-censored observations, upper values defining the left-censored observations, lower values defining the right-censored observations, the intervals defining the interval-censored observations, and F the cumulative distribution function of the parametric distribution Helsel (2005).
As fitdist
, fitdistcens
returns the results
of the fit of any parametric distribution to a data set as an S3 class
object that can be easily printed, summarized or plotted. For the
salinity
data set, a lognormal distribution or a
loglogistic can be fitted as commonly done in ecotoxicology for such
data. As with fitdist
, for some distributions (see Delignette-Muller et al. (2014) for details), it is necessary
to specify initial values for the distribution parameters in the
argument start
. The plotdistcens
function can
help to find correct initial values for the distribution parameters in
non trivial cases, by a manual iterative use if necessary.
fsal.ln <- fitdistcens(salinity, "lnorm")
fsal.ll <- fitdistcens(salinity, "llogis", start = list(shape = 5, scale = 40))
summary(fsal.ln)
## Fitting of the distribution ' lnorm ' By maximum likelihood on censored data
## Parameters
## estimate Std. Error
## meanlog 3.3854 0.06487
## sdlog 0.4961 0.05455
## Loglikelihood: -139.1 AIC: 282.1 BIC: 287.5
## Correlation matrix:
## meanlog sdlog
## meanlog 1.0000 0.2938
## sdlog 0.2938 1.0000
summary(fsal.ll)
## Fitting of the distribution ' llogis ' By maximum likelihood on censored data
## Parameters
## estimate Std. Error
## shape 3.421 0.4158
## scale 29.930 1.9447
## Loglikelihood: -140.1 AIC: 284.1 BIC: 289.5
## Correlation matrix:
## shape scale
## shape 1.0000 -0.2022
## scale -0.2022 1.0000
Computations of goodness-of-fit statistics have not yet been
developed for fits using censored data but the quality of fit can be
judged using Akaike and Schwarz’s Bayesian information criteria (AIC and
BIC) and the goodness-of-fit CDF plot, respectively provided when
summarizing or plotting an object of class fitdistcens
.
Functions cdfcompcens
, qqcompcens
and
ppcompcens
can also be used to compare the fit of various
distributions to the same censored data set. Their calls are similar to
the ones of cdfcomp
, qqcomp
and
ppcomp
. Below are examples of use of those functions with
the two fitted distributions to the salinity
data set (see
Figure @ref(fig:cdfcompcens)). When qqcompcens
and
ppcompcens
are used with more than one fitted distribution,
the non uniqueness rectangles are not filled and a small noise is added
on the y-axis in order to help the visualization of various fits. But we
rather recommend the use of the plotstyle
ggplot
of qqcompcens
and
ppcompcens
to compare the fits of various distributions as
it provides a clearer plot splitted in facets (see
?graphcompcens
).
par(mfrow = c(2, 2))
cdfcompcens(list(fsal.ln, fsal.ll), legendtext = c("lognormal", "loglogistic "))
qqcompcens(fsal.ln, legendtext = "lognormal")
ppcompcens(fsal.ln, legendtext = "lognormal")
qqcompcens(list(fsal.ln, fsal.ll), legendtext = c("lognormal", "loglogistic "),
main = "Q-Q plot with 2 dist.")
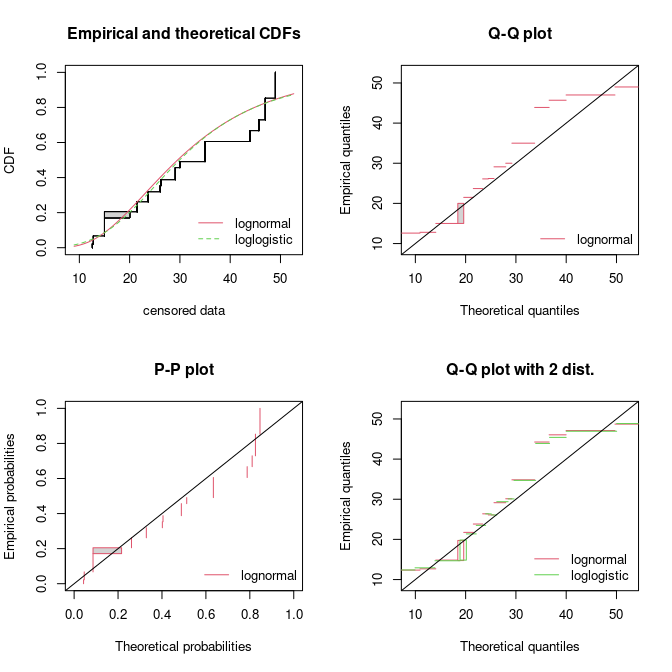
Some goodness-of-fit plots for fits of a lognormal and a loglogistic
distribution to censored data: LC50 values from the
salinity
data set.
Function bootdistcens
is the equivalent of
bootdist
for censored data, except that it only proposes
nonparametric bootstrap. Indeed, it is not obvious to simulate censoring
within a parametric bootstrap resampling procedure. The generic function
quantile
can also be applied to an object of class
fitdistcens
or bootdistcens
, as for continuous
non-censored data.
In addition to the fit of distributions to censored or non censored
continuous data, our package can also accomodate discrete variables,
such as count numbers, using the functions developped for continuous
non-censored data. These functions will provide somewhat different
graphs and statistics, taking into account the discrete nature of the
modeled variable. The discrete nature of the variable is automatically
recognized when a classical distribution is fitted to data (binomial,
negative binomial, geometric, hypergeometric and Poisson distributions)
but must be indicated by fixing argument discrete
to
TRUE
in the call to functions in other cases. The
toxocara
data set included in the package corresponds to
the observation of such a discrete variable. Numbers of Toxocara
cati parasites present in digestive tract are reported from a
random sampling of feral cats living on Kerguelen island (Fromont et al.
2001). We will use it to illustrate the case of discrete
data.
## 'data.frame': 53 obs. of 1 variable:
## $ number: int 0 0 0 0 0 0 0 0 0 0 ...
The fit of a discrete distribution to discrete data by maximum
likelihood estimation requires the same procedure as for continuous
non-censored data. As an example, using the toxocara
data
set, Poisson and negative binomial distributions can be easily
fitted.
(ftoxo.P <- fitdist(toxocara$number, "pois"))
## Fitting of the distribution ' pois ' by maximum likelihood
## Parameters:
## estimate Std. Error
## lambda 8.679 0.4047
(ftoxo.nb <- fitdist(toxocara$number, "nbinom"))
## Fitting of the distribution ' nbinom ' by maximum likelihood
## Parameters:
## estimate Std. Error
## size 0.3971 0.08289
## mu 8.6803 1.93501
For discrete distributions, the plot of an object of class
fitdist
simply provides two goodness-of-fit plots comparing
empirical and theoretical distributions in density and in CDF. Functions
cdfcomp
and denscomp
can also be used to
compare several plots to the same data set, as follows for the previous
fits (Figure @ref(fig:fittoxocarapoisnbinom)).
par(mfrow = c(1, 2))
denscomp(list(ftoxo.P, ftoxo.nb), legendtext = c("Poisson", "negative binomial"), fitlty = 1)
cdfcomp(list(ftoxo.P, ftoxo.nb), legendtext = c("Poisson", "negative binomial"), fitlty = 1)
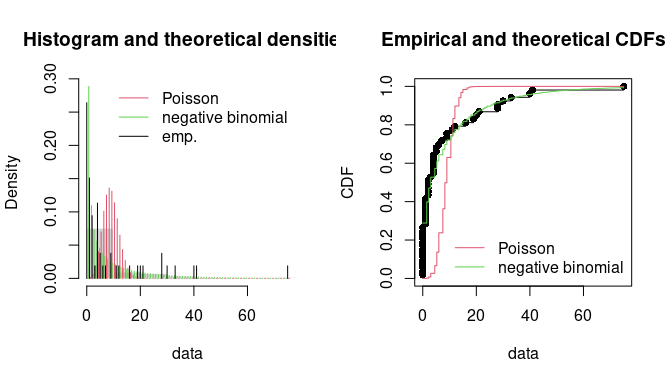
Comparison of the fits of a negative binomial and a Poisson distribution
to numbers of Toxocara cati parasites from the
toxocara
data set.
When fitting discrete distributions, the Chi-squared statistic is
computed by the gofstat
function using cells defined by the
argument chisqbreaks
or cells automatically defined from
the data in order to reach roughly the same number of observations per
cell. This number is roughly equal to the argument
meancount
, or sligthly greater if there are some ties. The
choice to define cells from the empirical distribution (data), and not
from the theoretical distribution, was done to enable the comparison of
Chi-squared values obtained with different distributions fitted on a
same data set. If arguments chisqbreaks
and
meancount
are both omitted, meancount
is fixed
in order to obtain roughly
cells, with
the length of the data set (Vose 2010). Using this default option the
two previous fits are compared as follows, giving the preference to the
negative binomial distribution, from both Chi-squared statistics and
information criteria:
## Chi-squared statistic: 31257 7.486
## Degree of freedom of the Chi-squared distribution: 5 4
## Chi-squared p-value: 0 0.1123
## the p-value may be wrong with some theoretical counts < 5
## Chi-squared table:
## obscounts theo Poisson theo negative binomial
## <= 0 14 0.009014 15.295
## <= 1 8 0.078237 5.809
## <= 3 6 1.321767 6.845
## <= 4 6 2.131298 2.408
## <= 9 6 29.827829 7.835
## <= 21 6 19.626223 8.271
## > 21 7 0.005631 6.537
##
## Goodness-of-fit criteria
## Poisson negative binomial
## Akaike's Information Criterion 1017 322.7
## Bayesian Information Criterion 1019 326.6
4. Conclusion
The R package fitdistrplus allows to easily fit distributions. Our main objective while developing this package was to provide tools for helping R users to fit distributions to data. We have been encouraged to pursue our work by feedbacks from users of our package in various areas as food or environmental risk assessment, epidemiology, ecology, molecular biology, genomics, bioinformatics, hydraulics, mechanics, financial and actuarial mathematics or operations research. Indeed, this package is already used by a lot of practionners and academics for simple MLE fits Voigt et al. (2014), for MLE fits and goodness-of-fit statistics Vaninsky (2013), for MLE fits and bootstrap Rigaux et al. (2014), for MLE fits, bootstrap and goodness-of-fit statistics (Larras, Montuelle, and Bouchez 2013), for MME fit Sato et al. (2013), for censored MLE and bootstrap Contreras, Huerta, and Arnold (2013), for graphic analysing in (Anand, Yeturu, and Chandra 2012), for grouped-data fitting methods (Fu, Steiner, and Costafreda 2012) or more generally Drake, Chalabi, and Coker (2014).
The fitdistrplus package is complementary with the
distrMod package (Kohl and Ruckdeschel 2010).
distrMod provides an even more flexible way to estimate
distribution parameters but its use requires a greater initial
investment to learn how to manipulate the S4 classes and methods
developed in the distr
-family packages.
Many extensions of the fitdistrplus package are planned in the future: we target to extend to censored data some methods for the moment only available for non-censored data, especially concerning goodness-of-fit evaluation and fitting methods. We will also enlarge the choice of fitting methods for non-censored data, by proposing new goodness-of-fit distances (e.g., distances based on quantiles) for maximum goodness-of-fit estimation and new types of moments (e.g., limited expected values) for moment matching estimation. At last, we will consider the case of multivariate distribution fitting.
Acknowledgments
The package would not have been at this stage without the stimulating contribution of Régis Pouillot and Jean-Baptiste Denis, especially for its conceptualization. We also want to thank Régis Pouillot for his very valuable comments on the first version of this paper.
The authors gratefully acknowledges the two anonymous referees and the Editor for useful and constructive comments. The remaining errors, of course, should be attributed to the authors alone.